3.8k
573
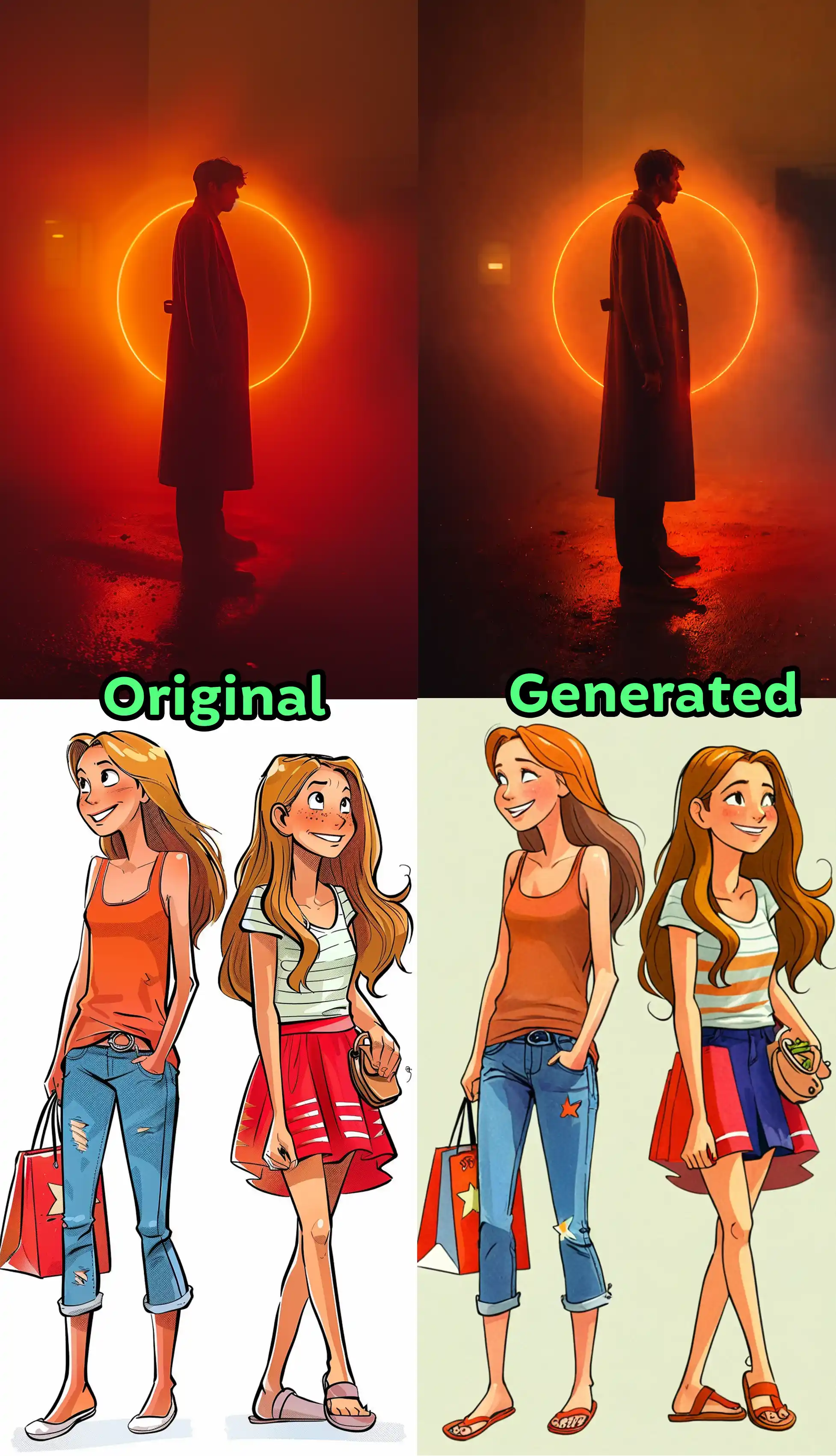
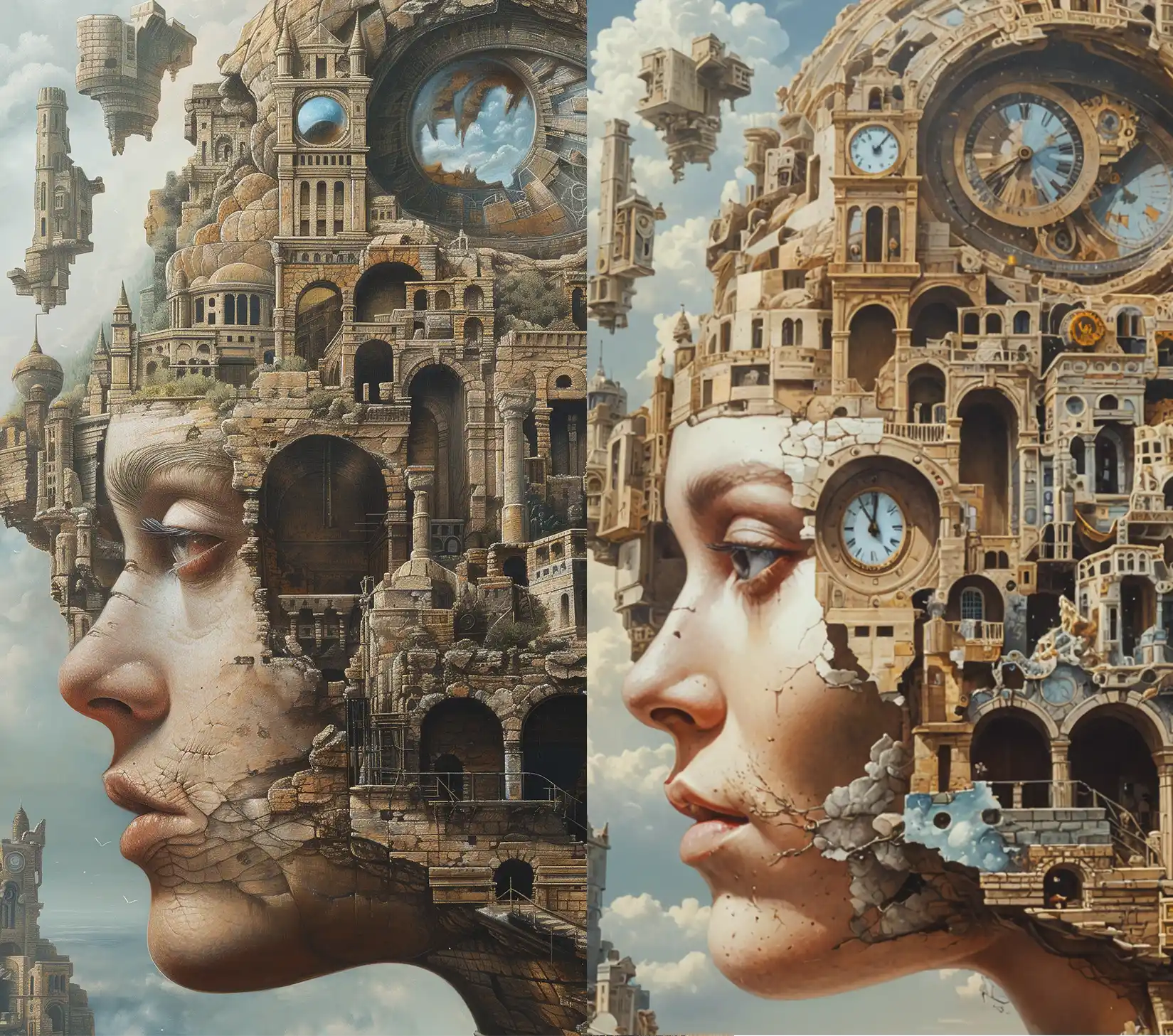
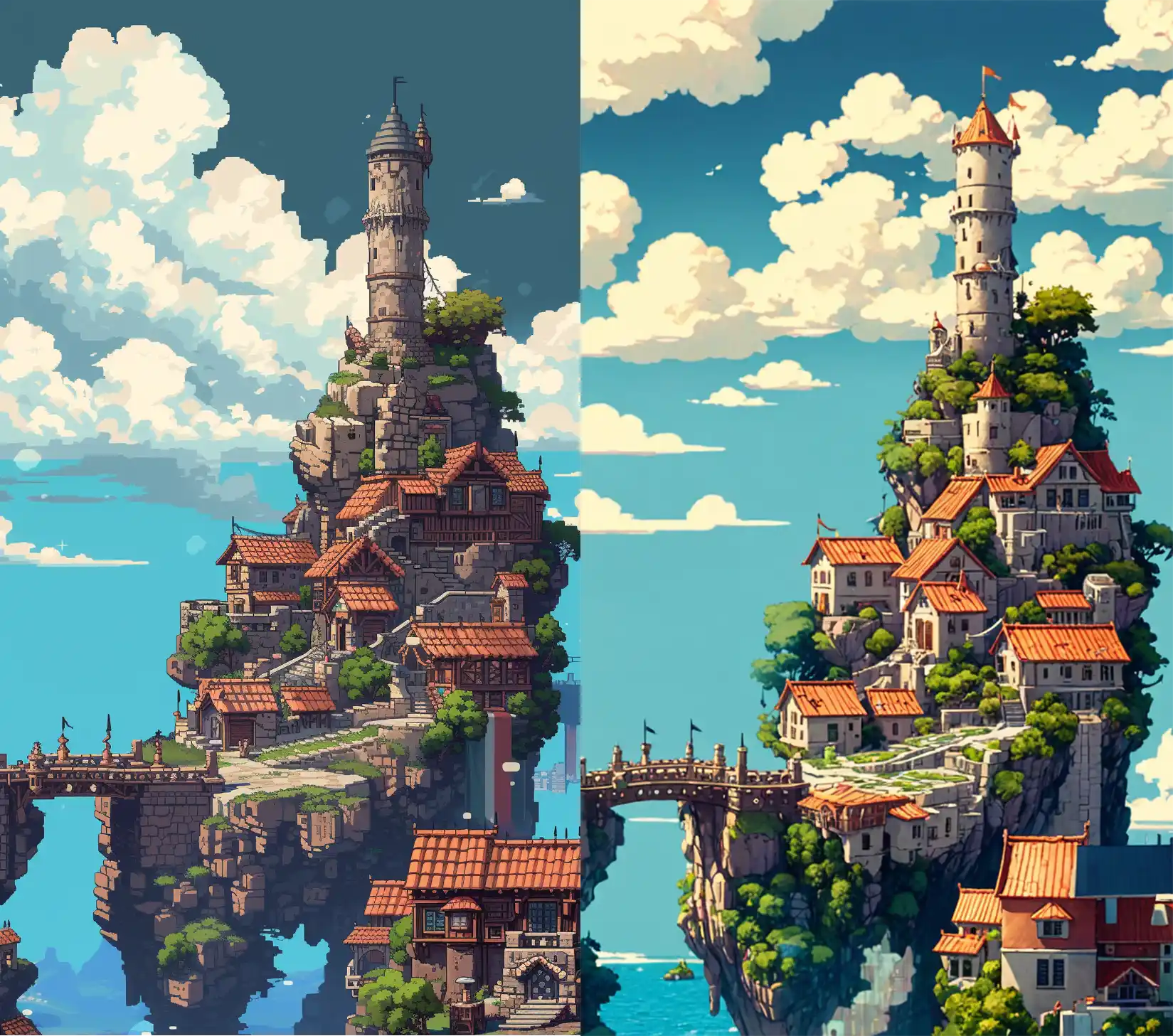
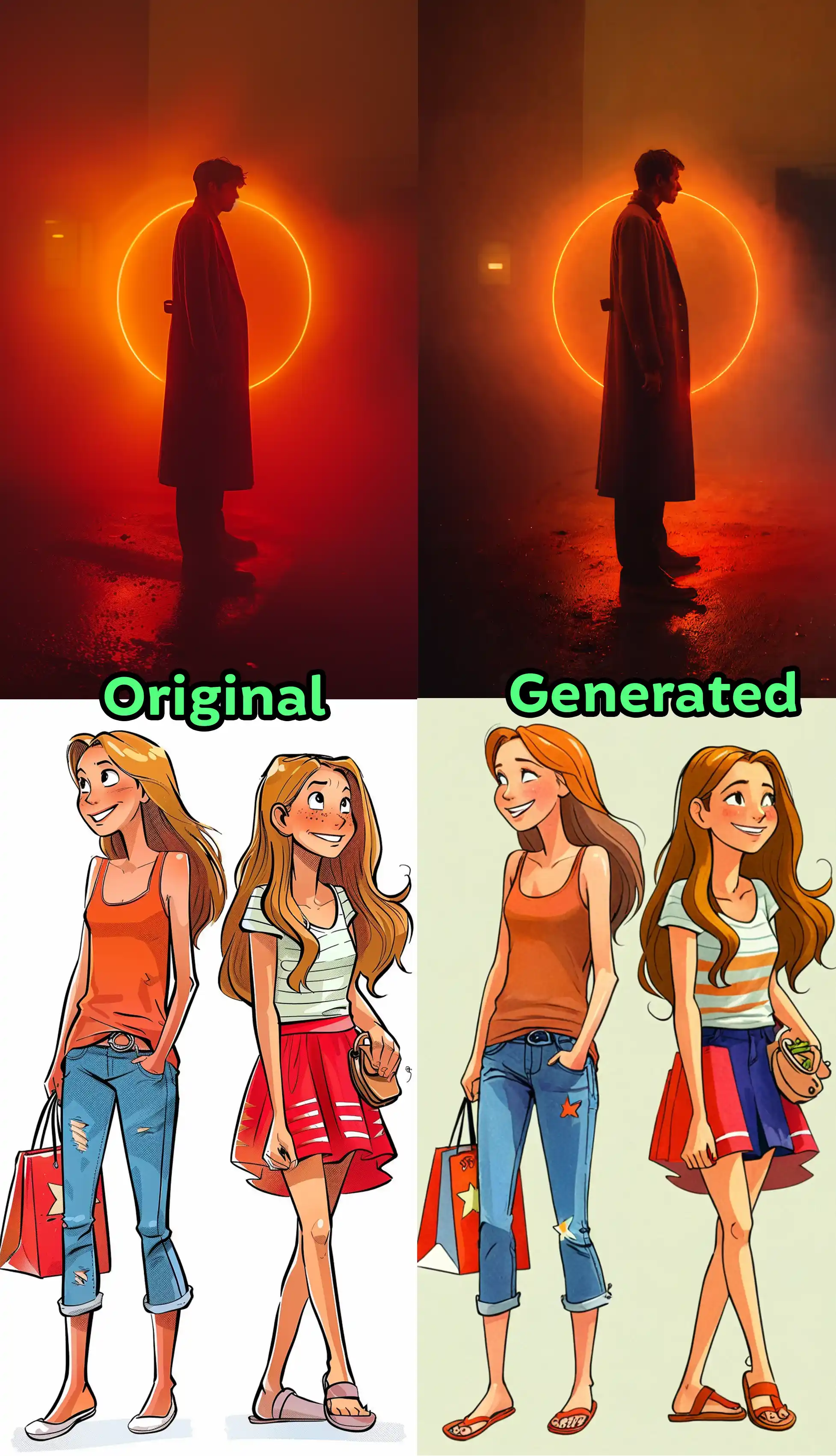
Hi everyone,
This workflow replicates the original image with immense accuracy. Let's break it down and understand how it works:
ControlNet with DepthAnythingV2: The new DepthAnythingV2 model is very powerful. This allows us to get the depth map of our original image so the generation can follow this precise mapping.
IPAdapter with "style transfer precise": IPAdapter allows us to replicate the style of the original image, but the newly developed "style transfer precise" method helps us reduce the bleeding in our final image, giving us more precise control.
Florence2: This is a bit unorthodox, but I really liked including it in this workflow. Simply put, Florence2 is an open-source vision model with many tricks, including masking, annotating, and captioning. In this workflow, I used the "more_detailed_caption" feature, which simply describes the image. Then I use this description as a CLIP Text Encoder's positive prompt.
Using these three methods, we guarantee that our final image will match the style, depth map, and prompt of the original.
Always remember, generation performance is related to your base model. For example, if you try to replicate an anime image and the result is not satisfactory, you should use an anime checkpoint.
*If the result does not satisfy you in terms of style, you can adjust the "weight" value of the IPAdapterAdvanced node or try other methods such as "strong style transfer" instead of "style transfer precise".
**You don't need to use Florence2; you can simply use your own manual CLIP Text Encoding method.
I would like to thank @Kijai and @Cubiq for developing these custom Comfy UI nodes at lightning speed and opening up many possibilities for us.
DepthAnythingV2: https://github.com/kijai/ComfyUI-DepthAnythingV2
IPAdapter Advanced: https://github.com/cubiq/ComfyUI_IPAdapter_plus/
Florence-2: https://github.com/kijai/ComfyUI-Florence2